Pytorch YOLO v5 Image Detection Bell Pepper
Github: https://github.com/EthanSeok/yolov5_detection
About YOLO
“이전의 물체 감지 프레임워크는 2-stage method (region proposal stage 와 classification stage)이었지만, YOLO는 one stage (single shot) 통합 물체 감지 모델로 개발되었다.”
“YOLO에서, 단일 CNN은 여러 bounding box와 클래스 확률을 동시에 예측할 수 있다. ‘Single Shot Detector’ SSD는 멀티스케일 학습을 위해 네트워크 내부의 다양한 수준의 피처 맵에서 앵커박스를 적용하여 객체를 매우 빠르고 정확하게 탐지하는 프레임워크이다.”
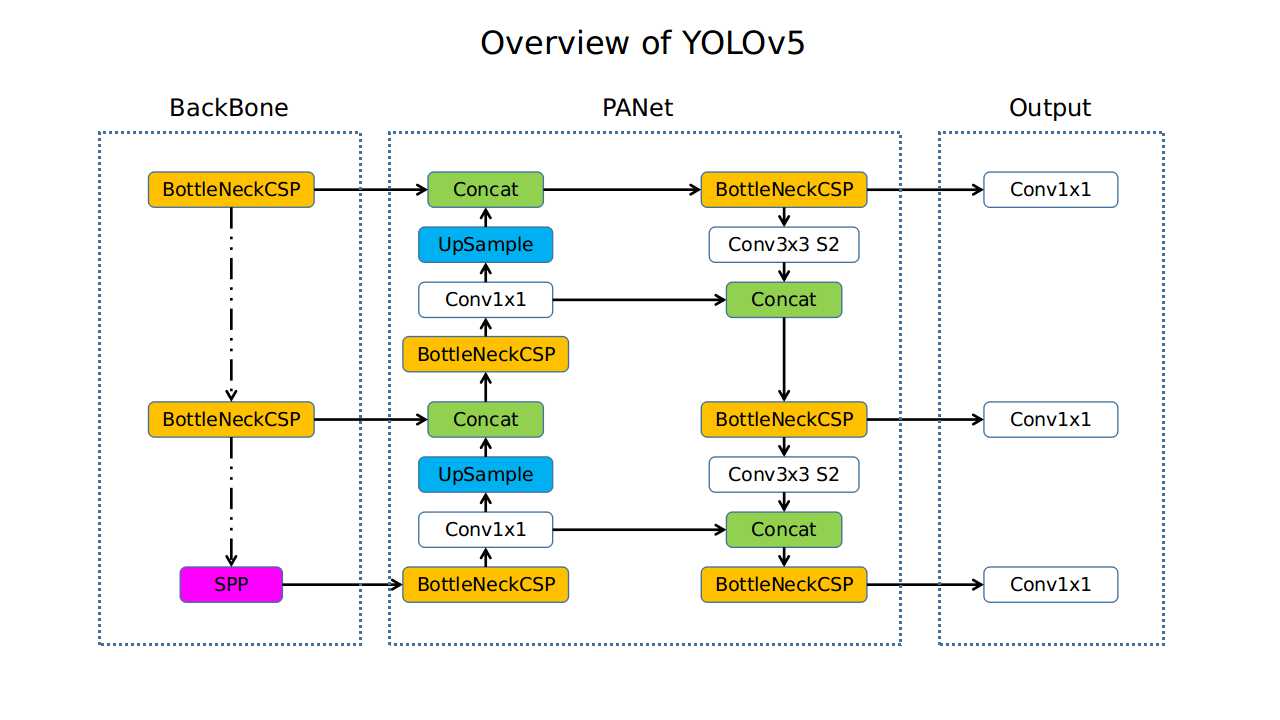
YOLO v5
- 직접 구득한 이미지 데이터 활용
- 과일의 객체 인식(Detection)
- Labelme를 이용한 클래스 어노테이션 - https://github.com/wkentaro/labelme
- RoboFlow를 통해 josn 파일을 txt 파일로 변경 후 학습
Labelme
컴퓨터 비전 인공지능 학습을 위한 어노테이션 데이터를 생성할 수 있는 라벨링 툴이다.
labelme
Labelme github 사이트: https://github.com/wkentaro/labelme
RoboFlow
Roboflow는 컴퓨터 비전(Computer Vision) 기술을 이용해 다양한 애플리케이션을 만들 수 있도록 지원해주는 서비스이다. 웹으로 구성되어 쉽게 사용이 가능하고, 주요한 특징은 무료 데이터셋을 제공하고, 사용자가 가지고 있는 데이터를 업로드하여 annotation을 할 수 있다.
LabelMe에서 어노테이션한 데이터는 json으로 저장되는데 YOLO v5에서는 json 데이터를 이용하지 못하기 때문에 이를 txt 파일로 변환해 주어야 한다. 이때, Roboflow가 json 어노테이션 파일을 업로드만 하면 txt 파일로 변환해 주고, 이를 다운받아 바로 적용할 수 있기 때문에
사용하기 좋은 사이트이다.
Roboflow 웹 사이트: https://roboflow.com/
어노테이션 json 이미지 업로드
labelme에서 생성한 어노테이션 json 파일 업로드
export 후 YOLO v5 선택
export에서 YOLO v5 선택 후 generate 하면 txt 데이터 생성됨
다운받은 파일 목록
압축 풀고 나온 파일은 YOLO 학습시 사용된다.
Wandb
wandb는 MLOps 플랫폼으로 머신러닝, 딥러닝을 학습하는데 필요한 다양한 기능들을 제공한다.
- 단 5줄의 코드로 추적, 버전 지정 및 시각화
- 모든 모델 체크포인트 재현
- 실시간으로 CPU 및 GPU 사용량 모니터링
wandb 지원 서비스
wandb를 이용해서 실시간 모델 학습 현황을 모니터링 할 수 있다.
실제 학습시 모니터링 화면
YOLO 학습 코드
wandb
설치
1 | !pip install wandb |
wandb
로그인
1 | !wandb login |
YOLO v5
git clone 후 설치
1 | %cd /content |
YOLO v5
학습시 필요한 라이브러리 설치
1 | %cd /content/yolov5/ |
1 | %cd / |
train test 데이터 분할
1 | from sklearn.model_selection import train_test_split |
yaml
파일 경로 지정 및 train test 데이터 지정
1 | import yaml |
모델 학습 코드
1 | cd /content/yolov5/ |
전체 학습 코드: https://colab.research.google.com/drive/1_AeNEQRcGnL5_m-uKQlX3vm7dPsboRCw#scrollTo=R_3ZsmewFzEc